Figure 1
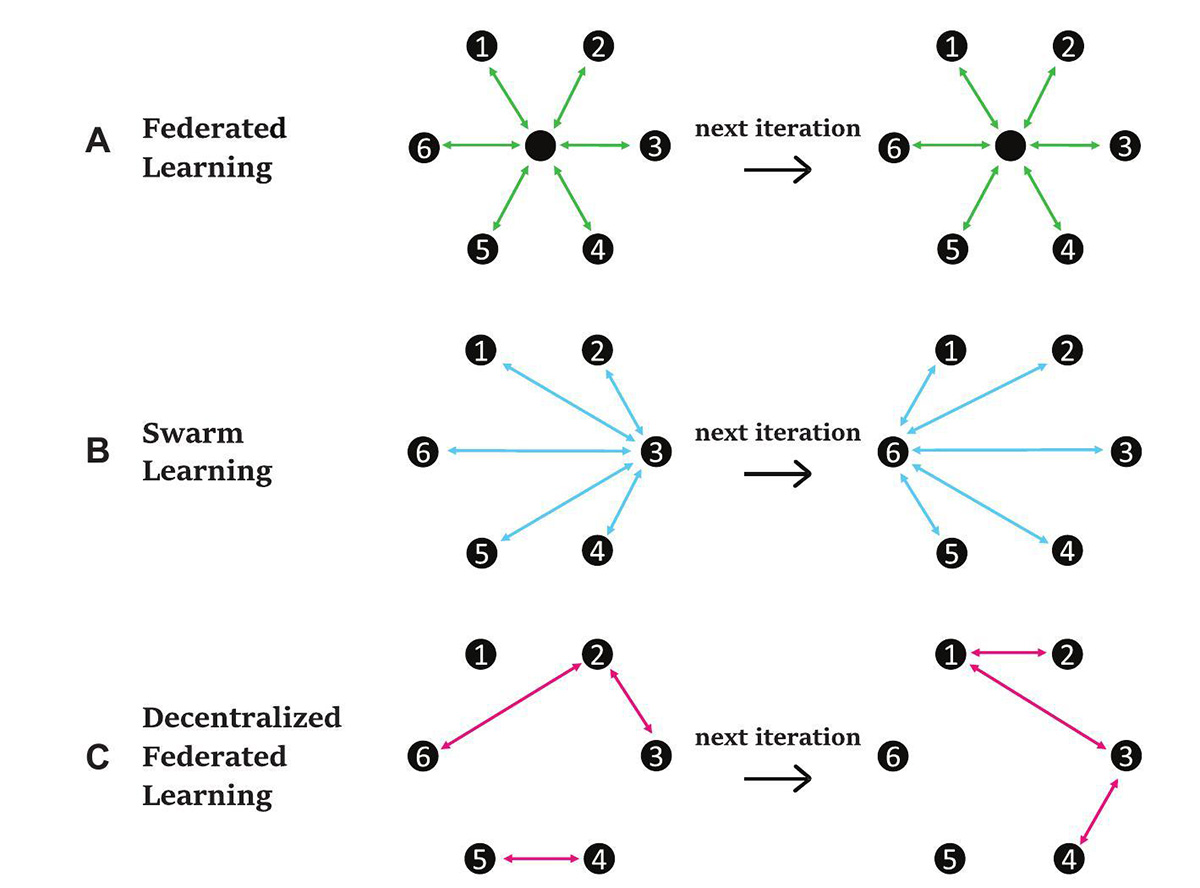
Download original image
Illustration of key concepts in different state-of-the-art federated learning frameworks. (A) Classical Federated Learning: a central client is needed to receive and transmit all essential information to other clients. It is equivalent to an all-to-all network without such a central center, i.e., every client in the network can receive information from all other clients. (B) Swarm Learning: there is no such a universal central client, but a potentially different central client is selected in every iteration. Mathematically, it is equivalent to FedAvg with varying central clients. (C) The proposed Decentralized Federated Learning: there is no need for a central client in any iteration. Any connected time-invariant or time-varying topology (under certain mild condition given in the Supplementary Information) has guaranteed performance, therefore unifying the classical federated learning and swarm learning.
Current usage metrics show cumulative count of Article Views (full-text article views including HTML views, PDF and ePub downloads, according to the available data) and Abstracts Views on Vision4Press platform.
Data correspond to usage on the plateform after 2015. The current usage metrics is available 48-96 hours after online publication and is updated daily on week days.
Initial download of the metrics may take a while.